Deep Dive into Product Analytics
Alright! Continuing with our series of blogs where we cover the AMAs we hold over our Slack channel.
Prabhu Konchada is Senior Product Manager at Apxor. Heading the product at one of India's fastest-growing product analysis. So here is our chance to have a masterclass in product analysis. No matter what type of product manager you are, analytics is something you will always look for!
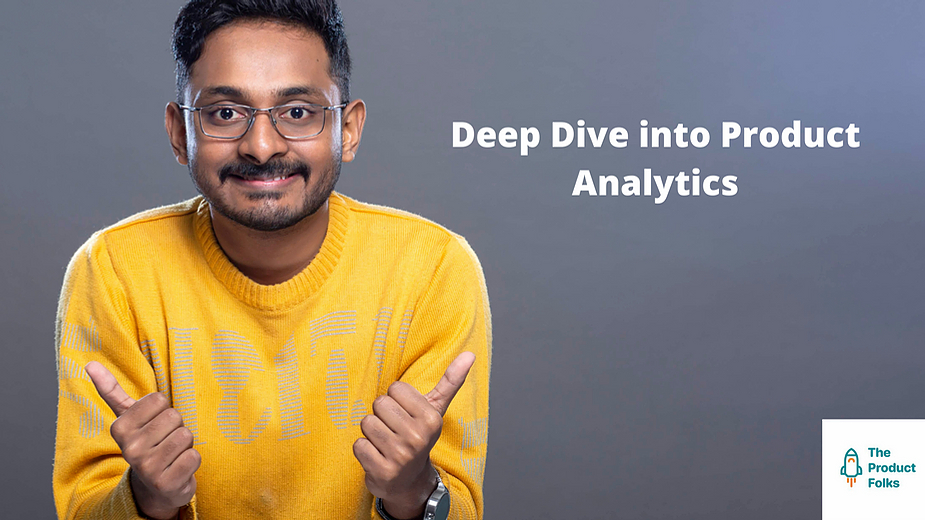
Here are some key takeaways:
Where to start with analytics when you cannot be sure about your hypothesis?
Let's take an example if the hypothesis to validate is if a feature is performing well / not. Quiz feature in the app we can start with looking at the adoption rate, are the adopted users contributing to product KPIs? Metric: % of new users who discovered that feature
Case: If adoption is good but not contributing to KPIs then the feature should be improved Looking at the funnels and analysing for drop-off reasons
Case: Adoption is not great but they are contributing to KPIs then the problem would be with adoption Should look at why users are not discovering? Is there a placement issue? OR Is it relevant to only a few particular segments of the audience? In case of very low adoption should look at CTRs of impressions to click rate to understand if there is a problem with prominence or they have noticed it but still not using it. From this drilling down to user research on the right sample helps
How to measure metrics to compare in your product for eg: metric differs from industry to industry?
Metrics which are not specific to the industry can be right away compared. Example: App launch time - whatsapp 1.2 sec, facebook 1.5-sec Metrics which are user-centric, Example: Engagement Index : DAU/MAU - Apptopia helps with engagement index, Example: How users are rating your product vs competitor product - play store console gives rating comparison
Any tools/strategies to know if your product analytics is wrong?
Is your instrumentation right ? Time & time I see this if data collection is not in place entire analytics falls flat, 'Event design' should be very clear covering the cases. Are the events unambiguous? Ex : ScreenLaunched is logged from different places in the app and you cannot differentiateAre they triggered at the right time as expected? Ex: Payment Clicked can be logged on response vs button click- when logged on responses clicks would be a biased definition of metric in the tool? Ex : Week retention is taken as calendar day vs 24 hours window.Always better to compare the data of analytics tools to understand the gap in definitions between both
How do you balance data and intuition when making product decisions? What role does data play in making and justifying your decisions?
Data should help build better intuition / validate your hypothesis. We are responsible to ask better questions to hear our intuition which speaks at 3 decibels. Role data plays in decision making is like giving assurance to your decisions so that things are eliminated in whiteboard instead of the sprint board. Understanding the significance of the problem we are trying to solve in terms of how much impact it would have if it is launched. It helps to keep our biases away and keep everyone on the same page.
What's your decision making framework while categorizing product features as “must-have” and “nice-to-have”?
If it is the part of the main core loop that brings in a positive change in the primary KPIs it is a must-have. If we are aiming at supplementing the core loop and intended to improve the product KPIs it is good to have.
Can you explain your problem-solving technique (using data )
Example: There is a dip in the D1 retention trend- Is there any change in acquisition sources
If yes, any of the new acquisition sources are pulling the retention down (getting bad quality users) Measuring the activation rate, onboarding rate that impact retention. If nothing suspicious at the marketing level, is there any new release only in which the dip in retention can be observed? If yes, focus on changes made specifically to the release no, something has changed at your server-side (which is not specific to release) then debug the changes there any overdoing or underdoing of the external triggers (average push notifications, expired email campaigns etc)? probe into seasonal changes, content quality, etc will drill down on other external factors that happen outside the app.
How do you define which tool to use when? Say, a database query/power BI, clevertap or a google Analytics?
For daily monitoring & analysis, we can't run queries manually. This process cannot be scaled so for all the frequent analysis types we have to go for a product analytics tool. 70-80% of the questions can be answered some or the other way using these tools. One time / infrequent or which are very specific to the domain that are not answered from tools can be queried.
What are the top 3 things to keep in mind while looking for data sets?
- Data understanding (right interpretation)
- Definition of metric in the tool
- Data sanity (correctness)
Without which there is always a possibility of getting to an incorrect conclusion.
How do you go about thinking about generic frameworks at Apxor so that your customers can solve their specific use cases?
Ability to do contextual EDA(exploratory data analysis) separates us from other frameworks. This helps our customers to work on the why problems, for example, # of dropoffs, page/screen at which they dropoff can be subjected to contextual diagnostic analysis to identify why users drop off.
Want to join the next conversation? We’ll be having another Product Chat soon, get your invite to our Slack community to get all the details. See you inside.